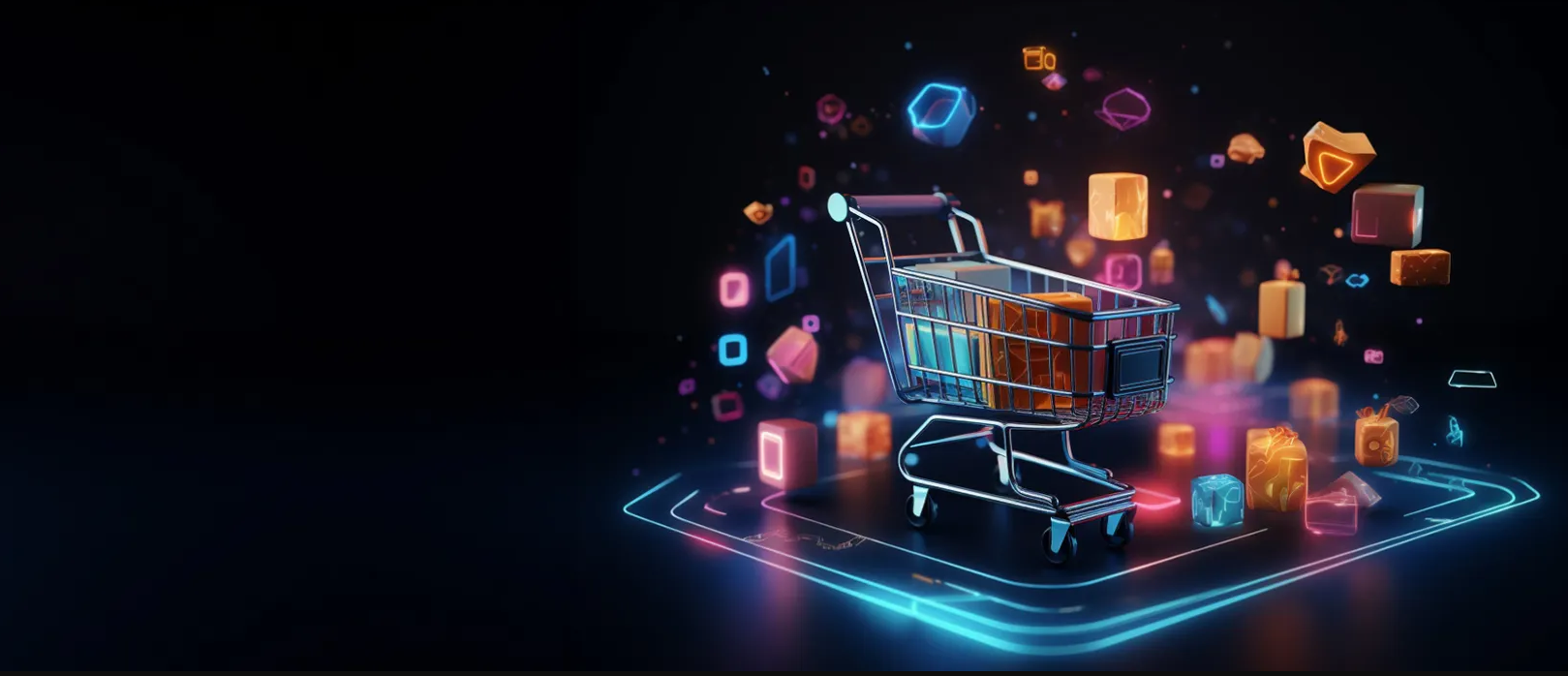
Understand the long-term value of your customers to make better-informed, short-term decisions on which customers to prioritize. In various business scenarios, encompassing marketing initiatives, refund processes, return policies, and loyalty programs, there exists an underlying assumption that customers possess a future value. However, in many instances, this prospective value remains unspecified, leading to considerable uncertainty surrounding critical decisions. These decisions may include determining the appropriate magnitude of discounts to offer, establishing thresholds for permissible returns, and deliberating whether to allow loyalty points to expire. In reality, the quantification of future customer value often proves challenging, contributing to the uncertainty inherent in decision-making processes. This ambiguity is underscored by the Pareto Principle, which posits that a significant portion of revenue—typically 80%—emanates from a minority of customers, constituting approximately 20% of the total customer base. Consequently, the identification and prioritization of high-value customers assume paramount importance in optimizing resource allocation and fostering sustained business success. By discerning which customers wield the greatest potential for long-term profitability, retailers can strategically allocate resources to cultivate and nurture these relationships. This strategic approach not only enhances customer satisfaction and loyalty but also bolsters revenue generation and fortifies the retailer's competitive positioning in the marketplace. Thus, by leveraging insights into customer value, businesses can chart a course toward sustainable growth and enduring success.
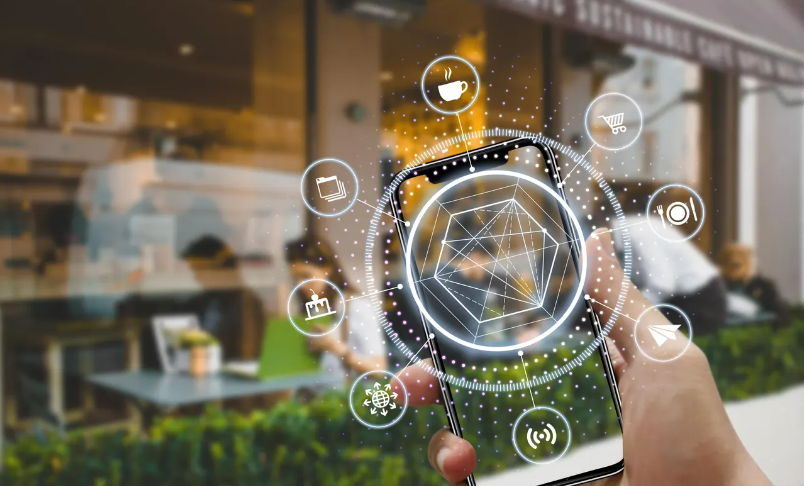
Retailers are increasingly leveraging data-driven methodologies to segment their customer base and discern the characteristics or demographic attributes that correlate with heightened overall value. However, while traditional approaches provide valuable insights at a macro level, the emergence of machine learning technologies presents an opportunity for a more nuanced and granular understanding of individual customer dynamics. With the integration of machine learning algorithms into their analytics frameworks, retailers can delve deeper into the vast troves of customer data at their disposal, unlocking actionable insights at an unprecedented level of detail. This enables retailers to move beyond broad customer segments and instead develop tailored profiles for each individual customer, capturing subtle nuances and preferences that might have previously gone unnoticed.
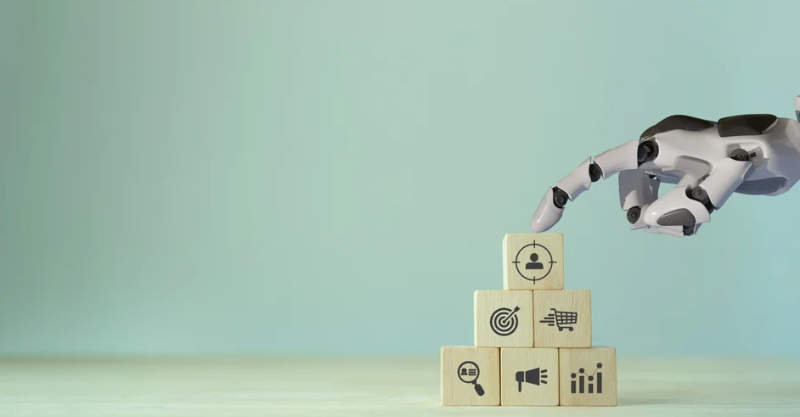
By employing machine learning algorithms, retailers can not only identify the specific factors that contribute to the future value of each customer but also generate precise estimations of their long-term worth to the business. These estimations encompass various dimensions, including anticipated purchase behavior, lifetime value, propensity to engage with promotions, and likelihood of churn. Armed with this comprehensive understanding of customer value, retailers can make informed decisions at a personalized level, balancing short-term costs and benefits against the broader strategic imperative of nurturing long-term customer relationships. This personalized approach extends across all facets of the retail operation, from targeted marketing campaigns and tailored product recommendations to individualized pricing strategies and customized loyalty programs. Moreover, the integration of machine learning-driven customer value estimations into decision-making processes empowers retailers to optimize resource allocation, allocate marketing budgets more effectively, and prioritize customer engagement initiatives based on their potential return on investment. By aligning their actions with the unique needs and preferences of each customer, retailers can cultivate stronger, more profitable relationships, driving sustainable growth and competitive advantage in today's dynamic retail landscape.
Copyright © 2023 Wizio AI