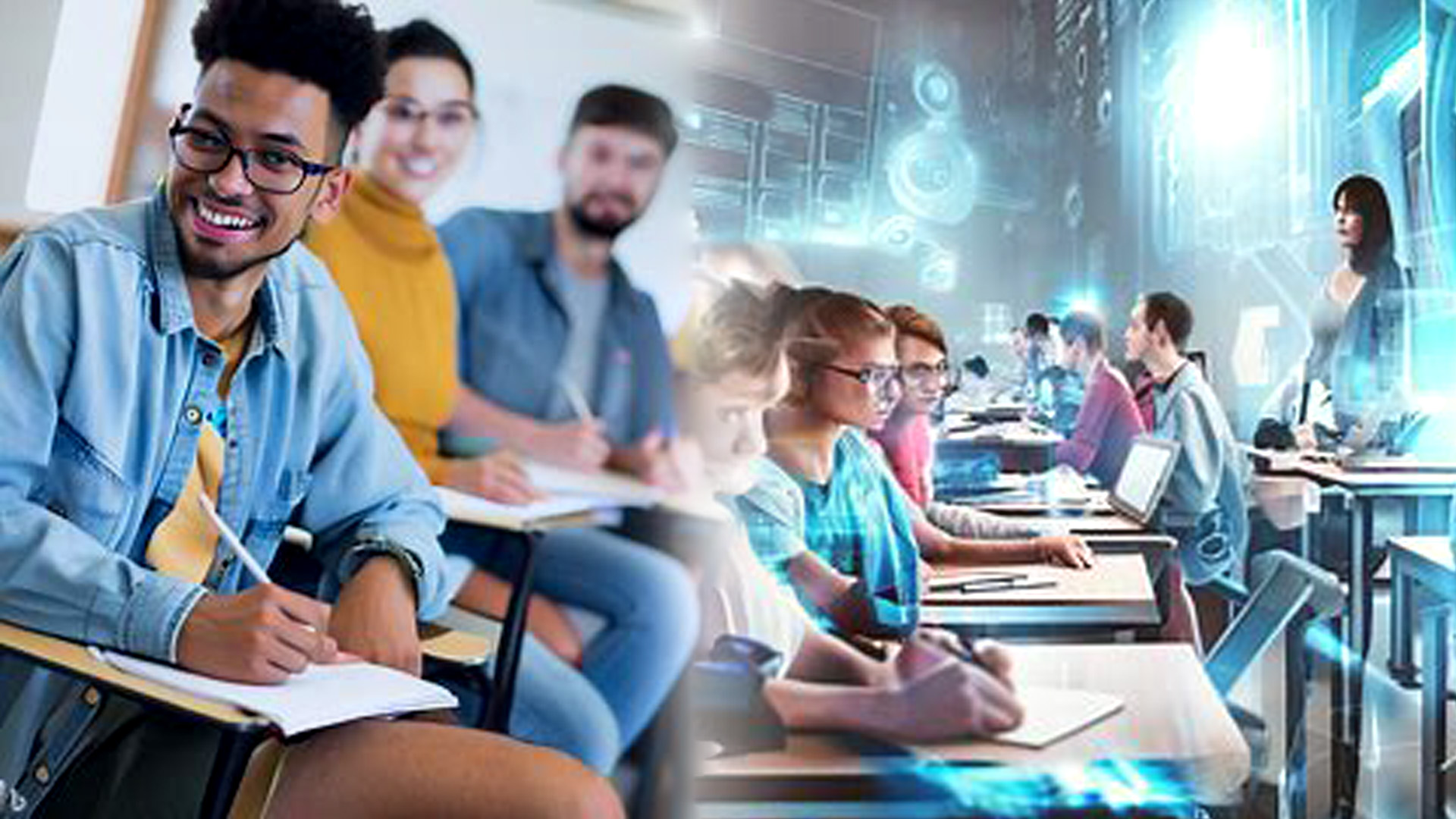
Predicting the likelihood that an accepted student will enroll (yield rate).
When universities and colleges embark on the rigorous process of selecting applicants for admission, they confront a multifaceted challenge with far-reaching implications. Central to this challenge is the concept of the yield rate, which stands as a crucial performance indicator for most academic institutions. The yield rate signifies the proportion of accepted students who ultimately choose to enroll in the institution. Remarkably, even prestigious universities and colleges often grapple with yield rates that hover below the 50 percent mark.
The intricacies of managing yield rates lie in the delicate balance that universities must strike between accepting an optimal number of students and ensuring that their incoming class aligns with institutional goals and standards. On one hand, if an institution accepts too many applicants, it risks overfilling its available spots, potentially straining resources and diluting the quality of the educational experience. On the other hand, accepting too few applicants may lead to an enrollment shortfall, where the institution fails to attract the desired caliber of students or achieve its desired class size.
To navigate this intricate terrain, universities must leverage sophisticated predictive modeling techniques to forecast the probability distribution of matriculation among the cohort of accepted students. This entails assessing each applicant's likelihood of enrolling based on various factors such as academic performance, extracurricular involvement, and fit with the institution's culture and values. Armed with these insights, admissions committees can make informed decisions about which applicants to admit, weighing the trade-offs between a student's level of interest and their probability of matriculating.
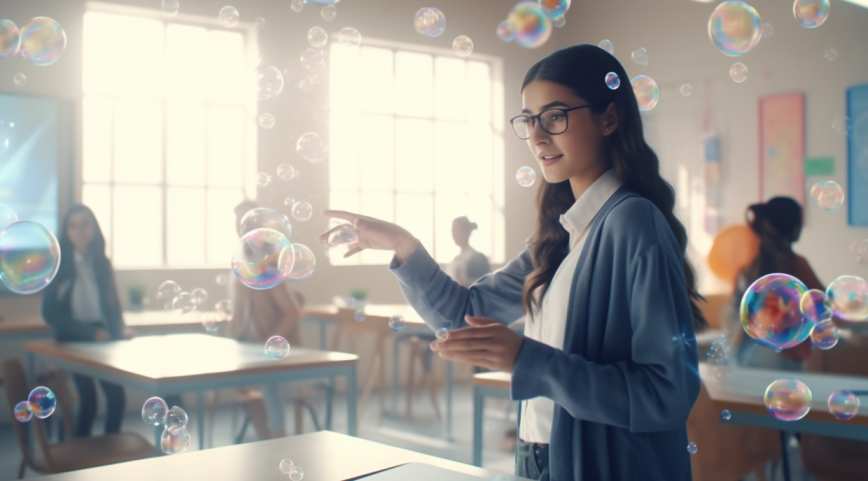
However, the complexities don't end there. Institutions with lower yield rates often find themselves compelled to accept a larger number of applicants to meet their enrollment targets, consequently leading to a higher acceptance rate. While a high acceptance rate may seem counterintuitive to notions of prestige, it becomes a necessary compromise to ensure that the incoming class is sufficiently populated. Yet, this strategic maneuvering underscores the delicate dance that universities must perform to balance their institutional goals with their reputation and standing in the academic community.
Moreover, the importance of accurate yield forecasting cannot be overstated. A faulty prediction can have far-reaching consequences, potentially resulting in missed revenue objectives, enrollment surpluses, or a mismatch between institutional capacity and student demand. Such outcomes not only jeopardize the financial stability of the institution but also tarnish its reputation and undermine its ability to attract top-tier talent in the future.
In essence, the pursuit of a higher yield rate is not merely a quest for numerical superiority but a strategic imperative that underscores the fundamental mission of academic institutions: to attract, educate, and empower the next generation of leaders and scholars. As such, achieving a delicate equilibrium between yield rates, acceptance rates, and precise forecasting methodologies is paramount to ensuring the long-term viability and success of the institution.
Utilizing the capabilities of artificial intelligence (AI) within your organization provides a transformative opportunity to enhance the predictive capabilities regarding the enrollment likelihood of accepted students. By leveraging advanced data analytics and machine learning algorithms, your university administrators and admissions officers can delve into the comprehensive dataset of prospective students, extracting valuable insights to forecast the probability of each accepted student ultimately enrolling.
This sophisticated predictive modeling enables your admissions team to move beyond the conventional approach of simply issuing acceptance letters to top-performing students. Instead, it empowers them to identify and prioritize candidates with the highest likelihood of enrollment, optimizing the institution's recruitment efforts and maximizing the yield from the accepted pool of applicants.
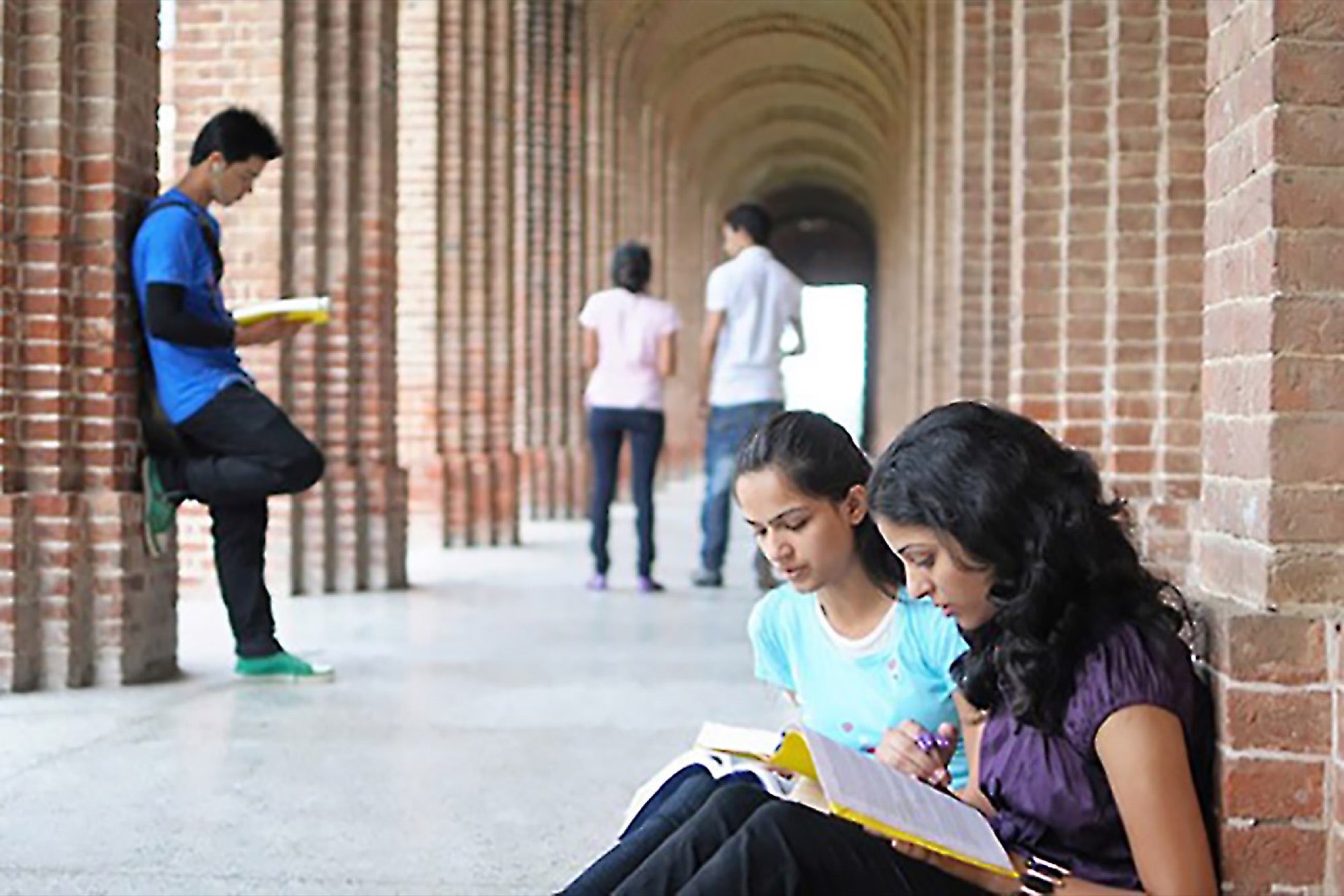
Furthermore, the implementation of AI-driven enrollment prediction systems offers a myriad of strategic advantages for your institution. Not only does it facilitate more targeted and efficient allocation of resources by accurately forecasting tuition revenues for the upcoming academic year, but it also enables your university to proactively anticipate staffing needs and plan various housing arrangements to accommodate the incoming student cohort effectively.
Moreover, by gaining insights into the predicted yield rate, your institution can extrapolate valuable information regarding the expected acceptance rates, providing critical intelligence to inform strategic decision-making processes. This holistic approach to enrollment management, underpinned by AI-driven predictive analytics, empowers your university to navigate the complexities of the admissions process with precision and foresight, ultimately enhancing operational efficiency and strategic planning capabilities.
In essence, the integration of AI technology into the enrollment forecasting process represents a pivotal step towards modernizing and optimizing your institution's admissions operations. By harnessing the power of data-driven insights, your university can unlock new opportunities to enhance student recruitment efforts, improve financial forecasting accuracy, and ultimately elevate the overall effectiveness and competitiveness of your academic programs.
Copyright © 2023 Wizio AI