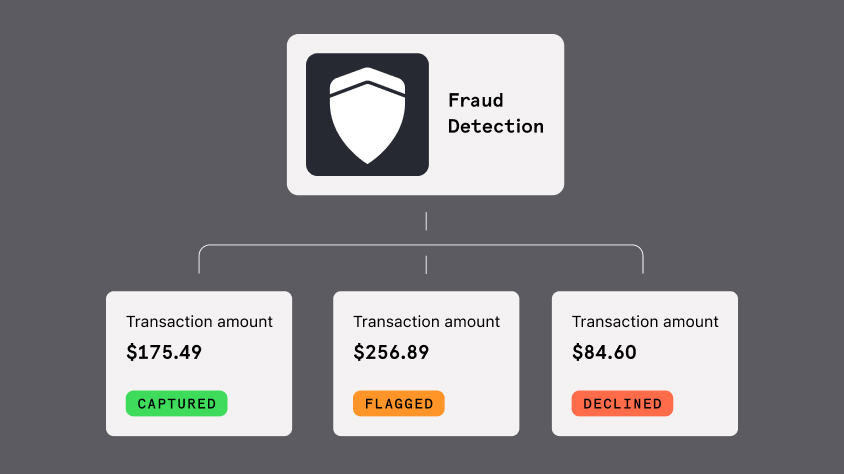
The annual cost of credit card fraud amounts to billions of dollars. By precisely forecasting potentially fraudulent transactions, banks can markedly decrease these unlawful activities, ensuring cardholders enjoy exceptional customer service.
Detecting and mitigating fraudulent transactions presents a significant challenge for financial organizations, given the substantial financial losses incurred and the potential reputational damage involved. However, conducting manual investigations for every transaction is not only prohibitively expensive but also inefficient, consuming valuable resources and time. Moreover, the risk of inadvertently subjecting innocent customers to scrutiny can result in a poor customer experience, leading to dissatisfaction and potential churn.
Recognizing these complexities, financial institutions are increasingly turning to artificial intelligence (AI) and machine learning techniques to streamline and enhance their fraud detection capabilities. By harnessing the power of AI, organizations can automate the process of building highly accurate predictive models that assess the likelihood of a transaction being fraudulent. This enables the creation of a sophisticated system wherein transactions are ranked according to their perceived risk, allowing fraud units to prioritize their investigative efforts effectively.
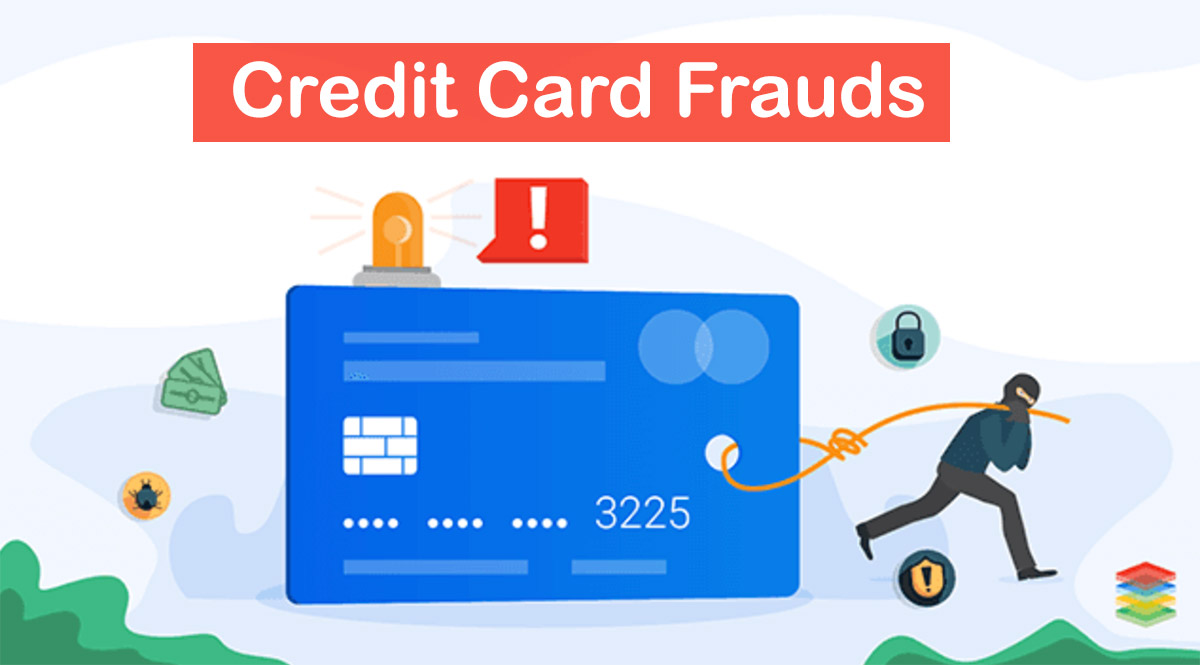
By implementing AI-driven fraud detection systems, financial organizations can achieve several benefits. Firstly, they can significantly reduce the incidence of fraudulent transactions, thereby minimizing financial losses and preserving the integrity of their operations. Secondly, by automating the detection process, they can optimize resource allocation and improve operational efficiency. Thirdly, by prioritizing suspicious transactions, they can ensure that investigative efforts are focused where they are most needed, enhancing the effectiveness of fraud prevention measures.
Furthermore, by providing a seamless and efficient customer experience, organizations can cultivate trust and loyalty among their client base. By leveraging AI technology to strike a balance between fraud detection and customer satisfaction, financial institutions can maintain a competitive edge in the market while safeguarding their customers' interests.
In summary, the integration of AI into fraud detection processes represents a transformative opportunity for financial organizations to enhance their risk management capabilities, minimize losses, and deliver superior customer experiences. By embracing innovative technologies and methodologies, they can stay ahead of emerging threats and adapt to evolving fraud schemes, thereby safeguarding their reputation and ensuring long-term success.
Artificial Intelligence (AI) serves as a pivotal tool in enhancing the effectiveness of fraud prevention systems by significantly refining the accuracy in predicting fraudulent transactions. This is achieved through a multifaceted approach that leverages sophisticated analytical techniques and machine learning algorithms.
One key aspect of AI-powered fraud prevention systems involves the utilization of supervised machine learning models. These models are trained on vast repositories of historical data encompassing past instances of fraudulent transactions. By meticulously analyzing the patterns and characteristics inherent in these data sets, the supervised models can discern subtle indicators of fraudulent behavior. Through iterative refinement and training, these models continuously learn and evolve, becoming increasingly adept at identifying potential instances of fraud in future transactions.
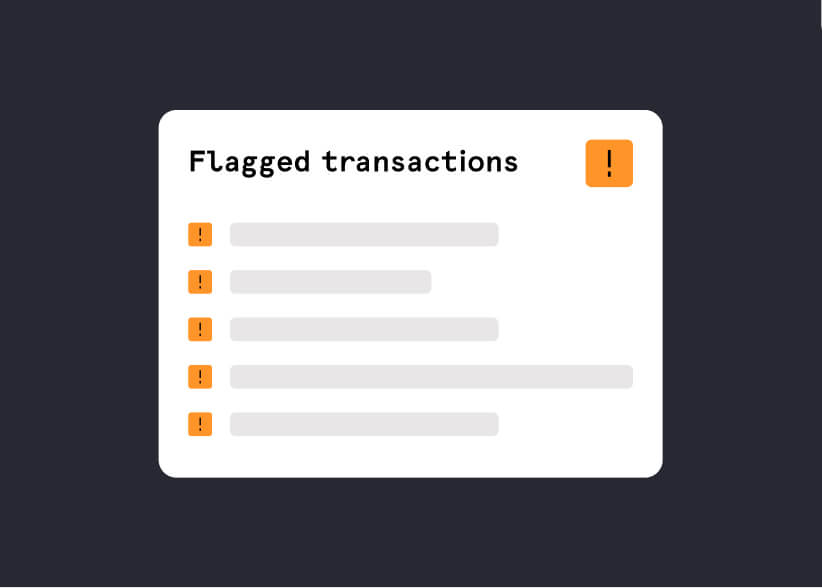
Furthermore, in scenarios involving previously unseen or novel fraudulent tactics, unsupervised machine learning models come into play. Unlike their supervised counterparts, unsupervised models do not rely on pre-labeled historical data for training. Instead, they employ advanced anomaly detection algorithms to flag transactions exhibiting deviations from established norms or patterns. These anomalous transactions are then subjected to manual review by fraud experts to determine their legitimacy.
Moreover, the dynamic nature of fraudulent activities necessitates ongoing adaptation and refinement of fraud prevention systems. As new fraudulent behaviors emerge, financial institutions must promptly update their detection mechanisms to effectively combat these evolving threats. This is facilitated through a feedback loop wherein newly identified fraudulent transactions are incorporated back into the training data for supervised models. By continuously exposing the models to real-world examples of fraudulent behavior, they can adapt and refine their predictive capabilities to accurately detect and thwart emerging fraud schemes.
Ultimately, the integration of AI into fraud prevention systems empowers financial institutions to significantly reduce the incidence of undetected fraudulent transactions. By leveraging advanced analytical techniques and machine learning algorithms, these systems can effectively identify suspicious activities while minimizing false positives. This not only safeguards the financial interests of institutions but also preserves the trust and confidence of customers by ensuring seamless and secure transaction experiences.
Copyright © 2023 Wizio AI